Disclaimer: Unless otherwise stated, opinions expressed below belong solely to the author.
Despite its fairly recent nascence, the consumer artificial intelligence (AI) industry is already in for a big shake-up if Meta’s chief AI scientist, Yann LeCun, is to be believed.
He shared his predictions during a technology debate at the recently concluded World Economic Forum at Davos, levelling criticism at generative AI and Large-Language Models (LLMs), which are behind the blowout rise of AI and currently power every popular consumer AI service, including ChatGPT, Gemini, Claude and Midjourney.
The four failures of (current) AI
LeCun, known for his scepticism of the current generation of AI models, pointed out that despite their usefulness, they fundamentally fail in four critical areas without which they can’t really transform the world or be capable of really outsmarting humans (or, in some ways, even animals).
Those four areas are:
- Awareness and understanding of the physical world
- Lack of persistent memory
- Lack of reasoning
- Inability of complex planning
LLMs have really only mastered a high (though not total) degree of understanding of the human language and are able to follow certain instructions mostly accurately, but are fundamentally driven by statistical probabilities after consuming large volumes of content.
They can write about most things, but they do not understand them since they are incapable of interfacing with the real world in any way.
They also lack the capacity to think or plan anything ahead, even though some attempts to push them in that direction are being made now, as evidenced by OpenAI’s recent launch of the Operator agent that can see your screen and take actions online for you.
Diffusion models, such as the ones used to generate images or videos when you use Midjourney, Dall-E or Sora, operate on similar principles, and this is where the lack of three-dimensional awareness has led to many embarrassing errors, like the classic example of people growing extra limbs or fingers.
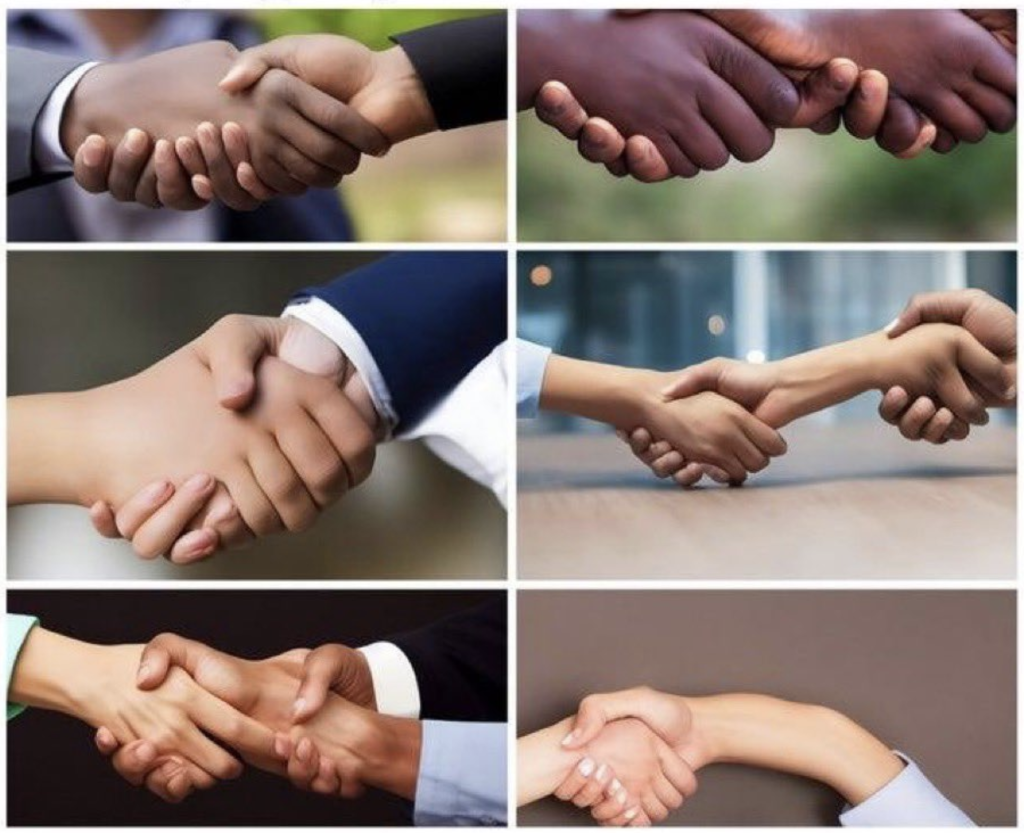
Because those models are not aware of how a real hand really looks like, what it does and how it functions in relation to the body, they aren’t capable of consistently generating accurate representations of it in different contexts. They merely approximate its appearance in line with the prompt provided by the user.
The understanding of the physical world by a cat is way superior to everything we can do with AI.
Yann LeCun
Just like LLMs, they have been fed enough data to be accurate and useful most of the time—but not all of the time. They are also incapable of reasoning about what they are actually doing.
In that sense, we’re not really in the age of artificial “intelligence” at all yet since the machines aren’t really thinking about what they are doing and do not have any actual knowledge of the world on the basis of which they would perform reasoning.
I think the shelf life of the current paradigm is fairly short [based on LLM], probably three to five years. I think within five years, nobody in their right mind would use them anymore, at least not as the central component of an AI system.
Yann LeCun
Billions down the drain?
It’s hard not to agree with LeCun on these fundamentals, although we have yet to see any alternatives to the models in use today.
It’s easy to point out that ChatGPT isn’t really thinking, but it’s much harder to produce an alternative that would do better than it does using the models developed by OpenAI.
However, it seems impossible for AI to have the revolutionary impact promised to us without addressing these core issues. A supremely accurate statistical algorithm can power a remarkably lifelike chatbot, but that doesn’t mean it is reasoning and taking conscious action.
It will be very useful, no doubt, as it already is in many areas, but it won’t take us anywhere near AGI or ASI.
This begs the question—are the hundreds of billions poured into current AI models a waste of resources?
After all, the current generation of AI has yet to pay for itself. OpenAI keeps bleeding money, and everybody in the industry relies on generous investor funding (or one’s own, given the deep pockets of Google, Microsoft or Meta).
But even as subsequent models get incrementally better, the reality seems to be that they are all built on the wrong foundations—and they cannot outgrow their limitations.
A model relying on a however complex set of probabilities will not start thinking all of a sudden. It is not equipped to think, remember or have any spatial awareness of the physical world. And it won’t achieve that no matter how much more data you feed it.
Large-Language Models carry this name for a reason. They were designed to process human language and are very good at it—so good that they are making us believe our computers are showing signs of thought.
But their magical behaviour is no different than the trick of a street illusionist.
Whether within five years or later on, once new models are developed, the current ones will end up in the dustbin of history as flawed but very convincing gimmicks that directed enormous amounts of capital into proper AI research.
Featured Image Credit: World Economic Forum